
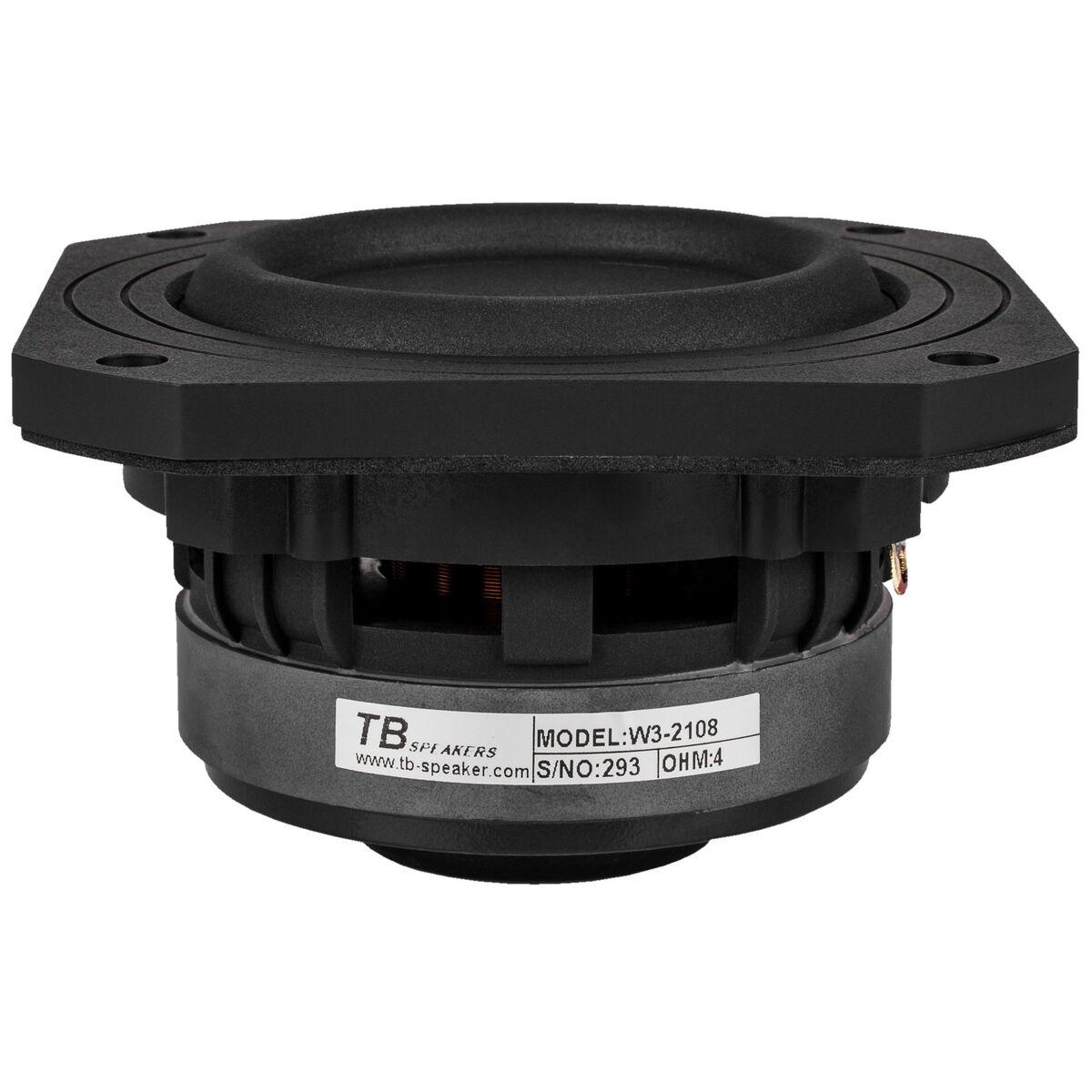
To date, there are many published reports on diameter-controlled growth of SWCNTs by chemical vapor deposition (CVD), the most popular synthesis method. In addition, control over SWCNT diameters is desirable for applications such as molecular transport 5, membranes 6, field emission 7, and sensing 8. A key property is the tube diameter, which is intimately related to its electronic properties 3, making diameter control of SWCNTs important for semiconducting device applications 4. The utilization of single-walled carbon nanotubes (SWCNTs) in a wide range of applications, including “Beyond Moore’s Law” computing 1, hinges on our ability to control their physical properties 2. Our study shows the promise for machine learning-driven diameter optimization and paves the way towards chirality-controlled SWCNT growth. Remarkably, our planner revealed significantly different synthesis conditions for maximizing the two diameter ranges in spite of their relative closeness. Extensive post-growth characterization showed high selectivity in the optimized growth experiments compared to the unoptimized growth experiments. We maximized two ranges corresponding to Raman radial breathing mode frequencies around 265 and 225 cm −1 (SWCNT diameters around 0.92 and 1.06 nm, respectively), and our planner found optimal synthesis conditions within a hundred experiments. not feasible and further optimized synthesis conditions to selectively grow SWCNTs within a narrow diameter range. Here we utilized a machine learning planner based on the Expected Improvement decision policy that mapped regions where growth was feasible vs. The diameters of single-walled carbon nanotubes (SWCNTs) are directly related to their electronic properties, making diameter control highly desirable for a number of applications.
